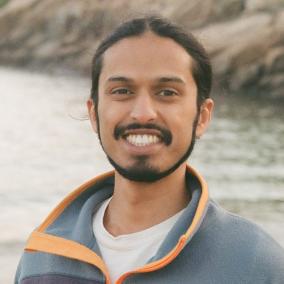
Advik Shreekumar
Thesis Writer
Research Fields
Behavioral Economics, Political Economy, EconometricsContact Information
Email Address
adviks@mit.edu
Research Fields
Behavioral Economics, Political Economy, Econometrics